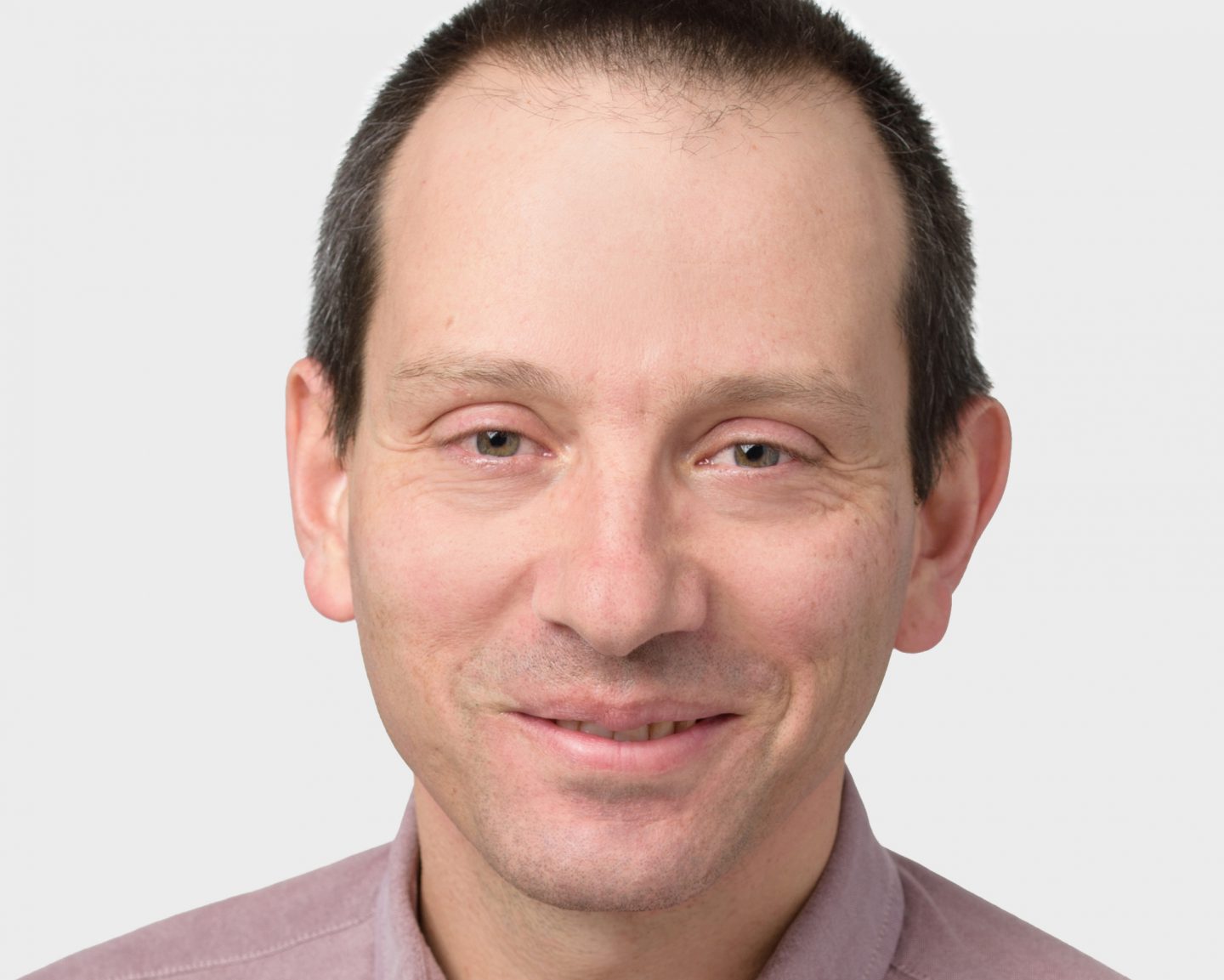
Speaker: Vitaly Shmatikov (Cornell Tech)
Modern machine learning models achieve super-human accuracy on tasks such as image classification and natural-language generation, but accuracy does not tell the entire story of what these models are learning. In this talk, I will look at today's machine learning from a security and privacy perspective, and ask several fundamental questions. Could models trained on sensitive private data memorize and leak this data? When training involves crowd-sourced data, untrusted users, or third-party code, could models learn malicious functionality, causing them to produce incorrect or biased outputs? What damage could result from such compromised models?
I will illustrate these vulnerabilities with concrete examples and discuss the benefits and tradeoffs of technologies (such as federated learning) that promise to protect the integrity and privacy of machine learning models and their training data. I will then outline practical approaches towards making trusted machine learning a reality.
14/12/2022 15:00, Aula 201, Palazzina D, Viale Regina Elena 295, Roma
Vitaly Shmatikov is a professor of computer science at Cornell Tech, where he works on computer security and privacy. Vitaly's research team has received the PET Award for Outstanding Research in Privacy Enhancing Technologies three times, as well as multiple Distinguished Paper and Test-of-Time Awards from the IEEE Symposium on Security and Privacy, USENIX Security Symposium, and the ACM Conference on Computer and Communications Security. Prior to joining Cornell, Vitaly worked at the University of Texas at Austin and SRI International.